Deep Learning in Spatial Applications and GIS
Machine learning has long been an indispensable tool in the realm of spatial analysis within Geographic Information Systems (GIS). From image classification to enriching data with clustering and modeling spatial relationships, machine learning has significantly advanced the capabilities of GIS tools over the decades. Within the vast landscape of machine learning, a powerful subset known as deep learning has emerged, revolutionizing the way computers understand and process spatial data.
Understanding Machine Learning and Deep Learning
Machine learning, a subfield of artificial intelligence (AI), utilizes advanced algorithms to effectively process structured data and tackle complex problem-solving tasks. Deep learning is a subset of machine learning characterized by the utilization of neural networks with three or more layers. “Deep” refers to the number of hidden layers in the neural network. Neural networks, which are designed to simulate the behaviour of the human brain, learn from large datasets and have proven to be instrumental in various applications. The network has the ability to engage in “end-to-end” learning, which enables it to process raw data and independently acquire knowledge about a particular task, such as classification. Deep learning has made remarkable advancements in recent times, even exceeding human performance in various tasks, including image object classification.
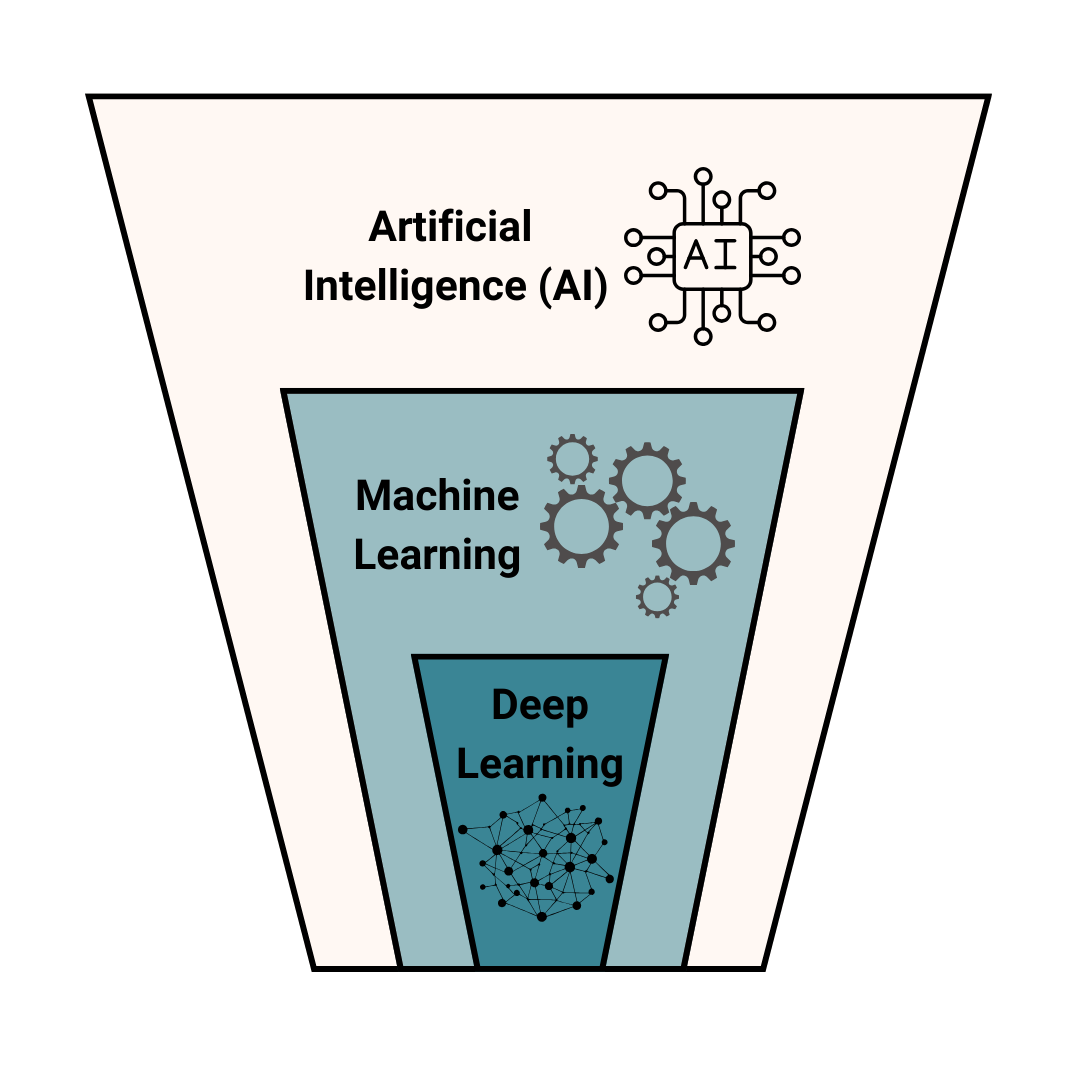
Deep Learning in Spatial Applications
Traditionally, GIS has relied on conventional machine learning tools, employing techniques like image classification and clustering to decipher spatial relationships. Deep learning is a big step forward for GIS systems because it allows them to understand and analyze spatial features on their own, without human intervention. This is particularly evident in situations where understanding spatial patterns is important for identifying complex objects like buildings, roads, or land cover types. A key strength of deep learning lies in its ability to efficiently process extensive sets of labeled data. In the context of GIS, this translates into the training of deep learning models with comprehensive datasets containing spatial information. For example, when classifying land cover types, a deep learning model can be trained using satellite imagery, enabling it to discern the unique features associated with various land cover classes.
Applications in GIS
The applications of deep learning in GIS are diverse and impactful. Image classification becomes more accurate as deep learning models can discern subtle differences between spatial features. Using clustering to enhance spatial data provides a more detailed understanding of spatial relationships. Deep learning is very useful for tasks like route optimization, urban planning, and environmental monitoring because it can model complex spatial patterns effectively.
Both ArcGIS and QGIS have developed toolsets for deep learning. Equator has developed an AI Digitizer tool that allows you to identify and digitize land cover types.
Conclusion
Deep learning has become a cornerstone in the field of spatial analysis, significantly elevating the capabilities of GIS tools. Its ability to automatically learn intricate features from large datasets, coupled with continuous improvement with more extensive data, makes deep learning a transformative force. With advancing technology, deep learning will likely have a more essential role in deciphering spatial data complexities and fueling innovation in different domains.